HybridML: Open source platform for hybrid modeling
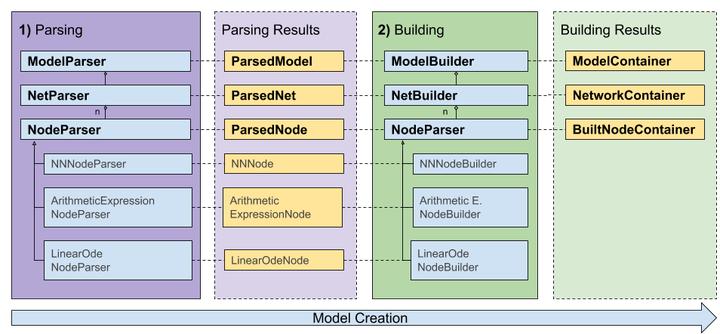
The combination of data-driven modelling with mechanistic model components, reduces the data demand and enables extrapolation of data-driven models.
However, building, training and evaluation of hybrid models is cumbersome with current frameworks. We developed HybridML, an open-source modeling platform, in which hybrid models can be trained, i.e., combinations of artificial neural networks, arithmetic expressions, and differential equations. We employ TensorFlow for artificial neural network training and Casadi to integrate ordinary differential equations and provide gradients of differential model equations enabling continuous time representations. HybridML provides also a JSON interface for the model development. We apply HybridML to an industrial case study, in which the trained model is used to predict drug concentrations over time, based on physiological information about the patients. To demonstrate its versatility, we also present a nonlinear application, where HybridML is used to model the spread of the COVID-19 pandemic in German federal states based on the state’s socio-economic attributes.
Open-source code
The open source code for HybridML is accessible here.
Applications
The proposed method has been used in an application to predict the concentration of the drug Fluvoxamine in patients’ blood over time. The input for the model is a set of covariates (attributes) such as gender, weight, age for each patient and the initial dose of Fluvoxamine given to that patient. Furthermore, a number of measurements of the Fluvoxamine concentration in the patients’ blood over time and the time since the patient took the medicine are provided for the estimation of the model parameters.
Key publications
- Merkelback, K., Schweidtmann, A., Mueller, Y., Schwoebel, P., Mhamdi, A., Mitsos, A., Schuppert, A., Mrzigold, T., Schneckener, S. (2022). HybridML: Open Source Platform for Hybrid Modeling. Computers & Chemical Engineering, In Press.