Hybrid modeling
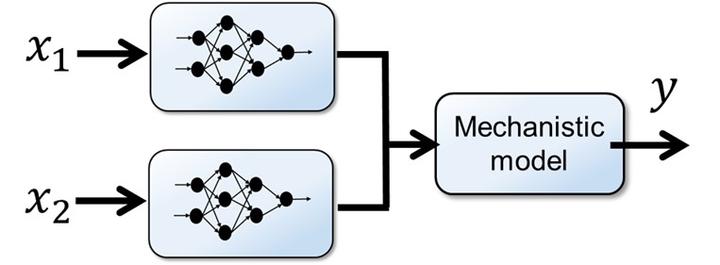
Integrating knowledge into AI is of utmost importance in chemical engineering.
Hybrid modeling combines mechanistic models with machine learning. For example, a combination of data-driven and mechanistic modeling can overcome the curse of dimensionality faced in purely data-driven approaches. Such hybrid modes also allow for extrapolation beyond the convex hull of the training data. However, the integration of knowledge into deep learning structures is still limited.
Key publications
-
Schweidtmann, A. M., Esche, E., Fischer, A., Kloft, M., Repke, J. U., Sager, S., & Mitsos, A. (2021). Machine Learning in Chemical Engineering: A Perspective. Chemie Ingenieur Technik.
-
Schäfer, P., Caspari, A., Schweidtmann, A. M., Vaupel, Y., Mhamdi, A., & Mitsos, A. (2020). The Potential of Hybrid Mechanistic/Data‐Driven Approaches for Reduced Dynamic Modeling: Application to Distillation Columns. Chemie Ingenieur Technik, 92(12), 1910-1920. [Schäfer et al., 2020]
-
Schweidtmann, A. M., Huster, W. R., Lüthje, J. T., & Mitsos, A. (2019). Deterministic global process optimization: Accurate (single-species) properties via artificial neural networks. Computers & Chemical Engineering, 121, 67-74. [Huster et al., 2020]
-
Rall, D., Schweidtmann, A. M., Kruse, M., Evdochenko, E., Mitsos, A., & Wessling, M. (2020). Multi-scale membrane process optimization with high-fidelity ion transport models through machine learning. Journal of Membrane Science, 608, 118208.