Generative artificial intelligence (AI) in chemical process engineering
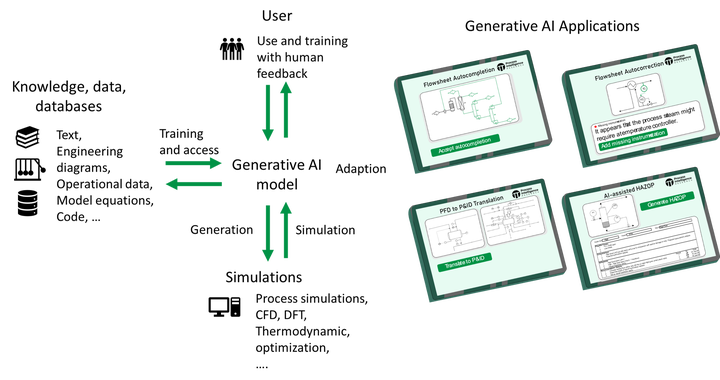
Generative artificial intelligence (AI) is transforming several sectors. This Comment provides a viewpoint outlining the potential significance of generative AI for chemical process engineering. Moreover, challenges for future research and development are outlined.
Generative artificial intelligence (AI) approaches are currently transforming various sectors. For instance, ChatGPT demonstrates impressive zero-shot performance for language generation. Likewise GitHub Copilot generates code, DeepL translates texts, Grammarly corrects texts, IBM RXN generates reaction outcomes, and Adobe Firefly generates images. The goal of generative AI models is to learn a parametric approximation of an underlying (unknown) data distribution. This allows to sample new data from the generative model mimicking the original data distribution. This technology is driven by a combination of big data, meaningful information representation, GPU computing, and modern deep learning architectures like transformers used in large language models. Besides the technology push, we are also in a situation with an exceptionally strong market pull. Big technology companies are investing heavily in generative AI as a considerable business potential is anticipated. Generative AI is believed to transform sectors and unleash the next wave of productivity. For example, McKinsey estimated in June 2023 that generative AI and other technologies have the potential to automate work activities that absorb 60 to 70 percent of employees’ time today.
In the context of chemical process engineering, generative AI is still in its infancy. In this Comment, I outline my vision for generative AI in chemical process engineering. I propose a few promising application areas for generative AI. Then, I discuss challenges and opportunities regarding future research and development.
I envision that generative AI will transform chemical process engineering in all aspects including modelling, optimization, design, control, and operation of process systems. As illustrated in the Figure, I foresee that the existing chemical engineering knowledge and data will become accessible through (domain- or even company-specific) foundation models. This access could be achieved through training but also through the ability of generative AI to write and execute queries to databases. Besides accessing existing data, generative AI models will also be able to interact with simulation and optimization environments. For example, generative AI algorithms will generate models, run simulations, and use the results to solve problems. The ability of generative AI to incorporate mechanistic knowledge and simulations has the potential to lead to the “next generation” of hybrid models. Moreover, the ability of generative AI algorithms to generate text, code, images, and more opens up new possibilities for user interaction and explanation of results.
Regarding the application side, I believe that there will be plenty of potential applications in process engineering. In this comment, I would like to briefly outline four potential process engineering applications: (1) autocompletion of flowsheets, (2) autocorrection of flowsheets, (3) P&ID generation, and (4), AI-assisted HAZOPs. In autocompletion, generative AI algorithms will autocomplete flowsheets including unit operations, topology, and design/operating variables. Such an algorithm could be integrated in simulation software. In autocorrection, generative AI algorithms will identify errors in P&IDs and other engineering documents and explain the users the suggested changes. This will not be limited to errors but will also include best practices to make processes more sustainable and safer. In P&ID generation, complete P&IDs will be generated based on Process Flow Diagrams (PFDs) and other relevant documents. In AI-assisted HAZOPs, generative AI will pre-fill HAZOP documents based on all available engineering documents, previous HAZOP reports, and (dynamic) simulations.